Story of Neighborhoods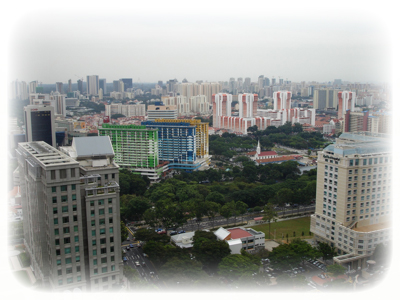
A neighborhood is a place built on identity. Neighborhoods [generally] conjure ideas of a geographic character. Some neighborhoods build identities through culture, age, and heritage. Others base their existence on location, a hill, a seaside or a seedy part of town. Each one provides a unique character with a story.
Neighborhood Map in Search
Internet services become more users focused; the need to provide greater localized content has expanded the requirements for more neighborhoods demographic information has also increased. Two key elements driving targeted localized interest are: consumer neighborhood name recognition and neighborhood demographic consistency. By supplying data based on defined locations with common characteristics –which consumers are familiar with-- companies and service organizations will be able to target these communities and their inhabitants more effectively.
•Descriptive Boundaries Method: Identifies street boundaries of neighborhoods using combination of data from city planning offices, chambers of commerce, and local real estate firms.
•Deduction Method: Uses satellite images to enhance estimation of neighborhood demarcations.
•Scatter Plot Method: Aggregates user survey responses to modify neighborhood boundaries over time, capturing evolution of neighborhoods caused by bleeding, where desirable or undesirable neighborhoods grow to slowly engulf surrounding areas.
Neighborhood datasets provide a meaningful refinement to understand locally correlated attributes, a significant improvement over currently used units such as census block groups and zip codes. Census block groups suffer from using fixed-quantity amalgamations of census blocks, units that may vary in size from individual city blocks all the way to large tracts of rural land. By arbitrarily combining census blocks, the ability to identify common attributes and trends in the grouping is severely diminished. This problem is also present in zip code divisions, which are chiefly determined by the availability of roadways for mail delivery, a factor that is only minimally related to group-wide attributes. On the other hand, our neighborhood data identifies groups that identify with each other, providing partitions that embody the strong associations between neighbors.
Our innovation enables application-oriented organization of information, allowing subjects of interest to be better partitioned and targeted. Data sets organized by neighborhood facilitate a bevy of applications across commercial, research, and governmental entities:
(1) Internet Granular Search. As the Internet proliferates, content is becoming increasingly personalized. Users are concurrently demanding and being empowered with the ability to easily access localized content relevant to them. Neighborhood datasets are an enabling extension of this trend. Search companies can employ this information to allow users to search based on a parameter to which they intrinsically identify: their neighborhood.
(2) Real Estate. Today real estate advertising in US newspapers is a $13 billion market (2006), but as an increasing number of homebuyers go online, spending will most likely shift to Internet advertisements as agents seek to target potential customers more effectively. Data shows that 80% of homebuyers in the US residential real estate market go online before they buy a home. Neighborhoods are an easy and convenient way to identify an area. Many premier real estate websites allow searching of listings by city or zip code, ideally people would like to search by neighborhood names. Though these parameters -- outside neighborhood names -- may be sufficient for an initial search, they are limited. Not only does a specific zip code often correspond to multiple (2 to 5) neighborhoods, but organizing listings by zip code is non-intuitive because home purchasing decisions are often based on attributes that are highly correlated by neighborhood, including home value, school district, and crime rate. As a result of these strong associations, neighborhoods are a natural partition for real estate companies to present their available listings (refer to Appendix C for example).
(3) Other. Despite the extensive list of other applications of neighborhood data, we provide only short descriptions in the interest of brevity:
Targeted Marketing. Neighborhood data aids advertisers to more efficiently pursue their intended segment by decreasing the costs necessary to market products or services to a desired audience.
Political Polling and Campaigning. The socioeconomic and ideological similarities among neighborhood denizens permit political groups to employ these datasets to target populations with specific viewpoints and values.
Public Health. The spatial locality of health outbreaks and the correlation between ethnic groups and specific genetic maladies, both evident in neighborhood-organized data, provide substantial guidance for policymakers seeking to manage and control these issues (refer to Appendix D for example).
Public Safety. Neighborhood boundaries emphasize stark delineations of the prevalence of crime in an area. More granular knowledge of these relationships directs a more effective deployment of existing public safety resources.
Various markets have already shown serious interest in neighborhood data. For example, in the search market, portions of our dataset have already earned us a licensing deal with Microsoft, and terms have been agreed to with both Yahoo! and Google. As for the real estate sector, a previous collaboration of a member of the management team with an innovative market player yielded robust growth for the online real estate company. Factle Maps also received enthusiastic responses from multiple lucrative markets coupled with the eagerness exhibited by other markets points to strong growth potential for this technology. We expect that many market landscapes are sufficiently mature for adoption of this technology already, let alone within 5 years.
Our early-entrant position in this market along with partnerships with the leading search companies punctuates our efforts to set the de facto standard for neighborhood data and create a “lock-in” effect. By design, our preeminence in a market as broad as the search market should encourage consumers in many other sectors to demand neighborhood-oriented data, which leads to diverse future licensing deals. We believe the search market will effectively act as a “beachhead” market to our entry into other markets such as real estate advertising (in a $146 billion annual market), insurance companies, polling, special interest groups (e.g. AARP), retail industry and city planning etc. The data which this technology generates is invaluable to all industries where understanding the composition and behavior of various neighborhoods will enable them to set their marketing strategy and reach specific customers directly.
The evolving role of the Internet as a replacement tool for traditional services coupled with the increased personalization and targeting of content by marketers leads directly to a market need for more granular, meaningfully organized data. The emergence of the Internet has already ushered once-ubiquitous services such as “snail mail” and newspaper classified advertising towards obsolescence; this trend will inevitably continue. Additionally, as more and more users assimilate search into their daily routine, they will have increasing demands for localized content; our technology contributes to satisfaction of these needs.
Besides US, Factle Maps will expand neighborhood identification services beyond US borders to Canada, Mexico, Europe, and eventually worldwide. We feel that early mastery of this significant contributing technology can assert and solidify our position in value chains across many industries.